CECL Implementation: Lessons Learned from First Adopters
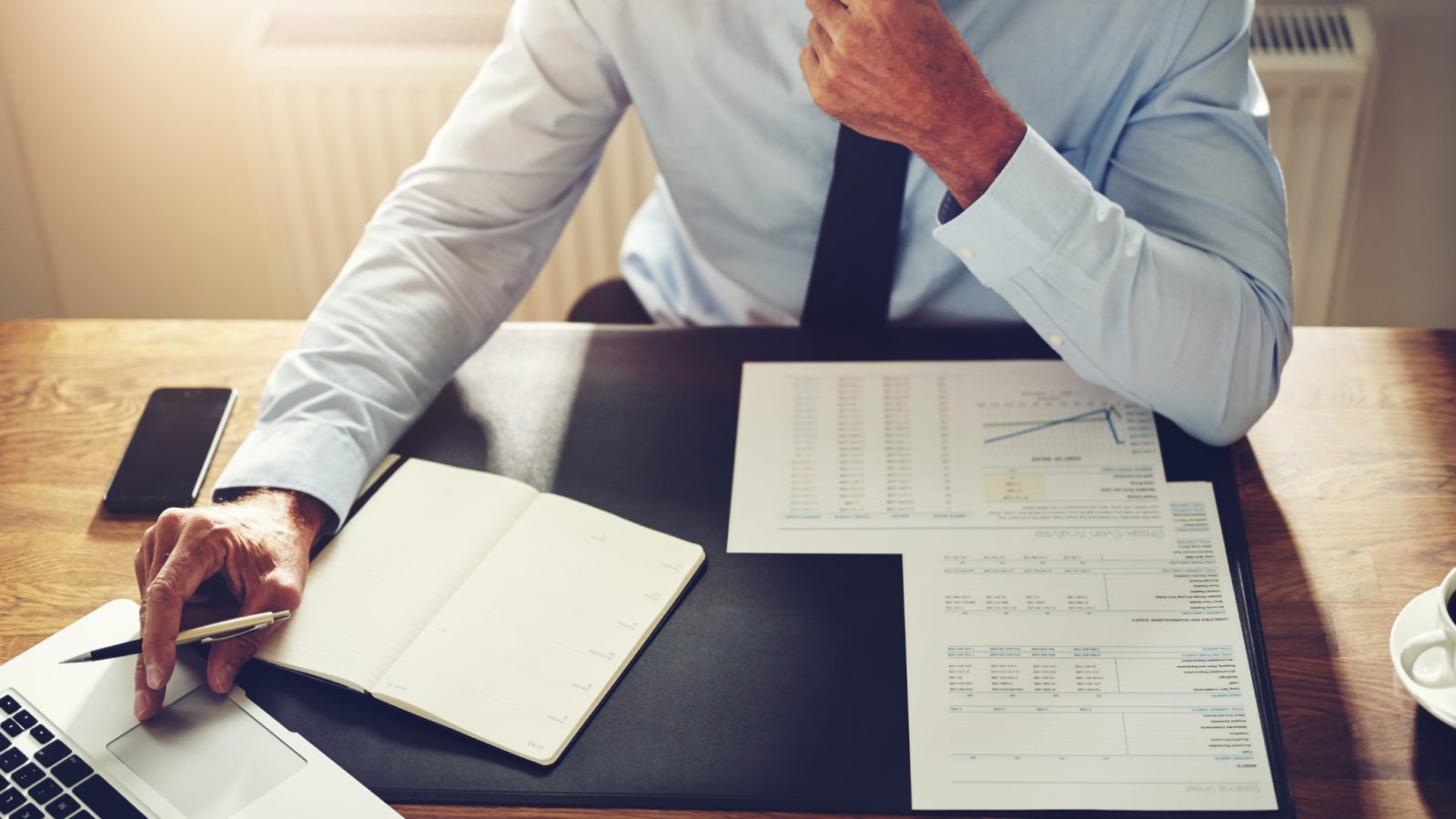
The past year was a year fraught with unprecedented challenges and changes impacting nearly all aspects of our lives. Given the truly world-changing impacts of the pandemic, implementation of the Financial Accounting Standards Board’s (FASB) current expected credit loss model, or CECL, was likely not at the top of your list of issues. However, for those institutions who were SEC issuers not classified as smaller reporting companies, CECL adoption and implementation did represent just one of the many hurdles to surmount during 2020.  Despite several other prominent pending accounting standards being delayed as a result of the pandemic, mandatory CECL adoption remains in effect for fiscal years beginning after December 31, 2022 for all other institutions at the time of this writing.
Fortunately for those who have not already adopted, we now have at least one year’s worth of data and insights from those who adopted in 2020 to draw from. Unsurprisingly, their number one recommendation remains: start early. For any readers who think they still have plenty of time to start the process, most adopters have stated the process took approximately two years, so the time to start is now if you have not already done so.
I’d like to first take a look at some of the trends and data we can glean from publicly available financial reports from those who adopted, and then discuss some of the lessons learned and best practices to keep in mind for institutions that are still in the planning stages. Given that the goal of CECL is to recognize the lifetime credit losses of a financial instrument at inception as opposed to the incurred loss model currently in place at most institutions, it’s no surprise that the allowance for credit losses (ACL) as a percentage of loans for financial institutions generally increased upon adoption of the new standard. Complicating this analysis is of course the fact that the COVID-19 pandemic also had a large impact on the ACL at most institutions as well. Fortunately, given the timing of the pandemic, we can attempt to somewhat isolate the impact of CECL adoption and the pandemic between the first quarter of 2020 and the remaining quarters. Generally, looking at public filings for several of the institutions who adopted on January 1, 2020, the ratio of the ACL to total loans increased on day 1 of adoption in the range of 10-40%, with the largest institutions generally having larger increases, perhaps unsurprisingly given their portfolio composition. These institutions tend to carry larger consumer loan and credit card portfolios than smaller institutions, which tend to generate larger lifetime losses, and therefore greater reserves are required under CECL. Generally, provisions in the following quarters during 2020 were significant, which we can assume was largely related to the anticipated and real effects of the pandemic. Looking at these figures, there is no doubt that CECL implementation has generally increased reserves, although the pandemic likely had the lion’s share of the effect over the course of the past year. In any case, given one of the stated goals of the standard is earlier recognition of losses, the numbers seem to suggest it’s working as intended.
Also worth noting is that the new standard does not prescribe any specific methodology in the estimation of the ACL. Of those that adopted, a wide variety of methodologies was chosen, ranging from the less complex loss rate methods to more complicated regression and discounted cash flow analyses. Generally, reviewing disclosures for the largest institutions shows that they are using the most complex modeling techniques and are also making use of multiple models for different pools or portfolios. We would expect that for many smaller institutions, the use of a single, less complex model will be a reasonable approach.
With regard to methodology choice, one of the insights from the first round of adoptions is that data is king. We anticipate that the availability and reliability of data at an institution often will, and should, help drive the decision as to the type of model(s) used in estimating the ACL. Entities will need to evaluate the volume and quality of data available to them. Do you have loan level data spanning entire economic cycles readily available, or has a recent core software conversion or data loss event limited the amount of loan data to only the last few years? Even for entities who are fortunate enough to have loan level data spanning many years, were there effective internal controls in place throughout the historical period to ensure that data is accurate? Expect to be asked these questions by auditors and regulators. Building in time for data and model validation prior to your adoption date can help alleviate these concerns, and early adopters have indicated that data validation was a key step in the adoption process. Furthermore, one of the important lessons learned is not to put the cart before the horse in terms of choosing a methodology up front, only to find that the data available to you is not ideal for that methodology. For instance, an entity would need to consider the volume and quality of historical data regarding changes in risk rating status or past due status transitions before settling on a migration methodology.
So, what is an entity to do that does not have a sufficient wealth of data to draw from? In addition to choosing a less complex model, adopters augmented the data they did have internally with peer data. In some cases, more granular anonymized peer data is available from vendors; however, use of a vendor is certainly not required and there is plenty of publicly available historical peer data to draw from, at least on an aggregated basis. While use of peer data is entirely acceptable, there are two main points to keep in mind. First, we anticipate that regulators will expect the use of peer data to drop over time as an entity accumulates more of its own data necessary for its model. Second, expect some scrutiny from stakeholders including regulators and auditors as to why the peer data you chose was appropriate.
Another area of implementation where we anticipate there will be concern is in regard to pooling loans appropriately for the calculation. Time should be spent by institutions to carefully and thoughtfully segment loans into appropriate pools based on similar risk characteristics, but there are other factors to consider as well. Under the incurred loss model, institutions may have grouped loans into many different pools based on industry codes, loan types, risk ratings, etc. However, caution should be taken in just rolling those pools over to the CECL model, as pools with very few loans or a lack of sufficient historical data could result in highly sensitive segments which introduce a lot of volatility in the calculation. Also worth considering is whether the institution intends to utilize peer data in its calculation. If you intend to use call codes from historical call reports for peers for instance, this may be a limiting factor in the granularity of the pools. Remember, the new standard continues to state that if an asset doesn’t share risk characteristics with other assets, it can and should be evaluated individually.
The quantitative side of the allowance for credit losses calculation gets the most attention; however, also important and new under the CECL standard is the incorporation of reasonable and supportable forecasts into the model. Qualitative factors are still in play and entities should be cautious as to not simply duplicate qualitative factors in the forecast. Generally, qualitative factors utilized are similar to those utilized in the incurred loss model. In considering whether an adjustment represents a qualitative adjustment versus a forecast adjustment, entities should consider the duration of the impact. Generally, a long lasting change like an alteration to an institution’s risk rating system would continue to be a qualitative adjustment affecting a loan pool from the time the change was made long into the future, whereas temporary changes attributed to current expectations about the near future represent forecast period adjustments. What many observers have noted in relation to forecasting is that the forecast period has shortened. This is simply a recognition of human inability to predict the future, likely made all the more clear by the onset of the pandemic. While initially, many in the industry predicted 3 to 5 year timelines for a forecast period, the majority of adopters are utilizing shorter forecast periods, in the 1 to 2 year range, either with an immediate reversion to historical averages or a straight-line reversion over a period of time. Predicting macroeconomic trends far into the future is something very few human beings have been able to do effectively, and is probably not something your institution wants to be in the business of doing.
Speaking of qualitative factors and forecasting: as has always been the case when judgment is involved, documentation is critical. Most early criticism has come related to not having sufficient documentation versus the actual level of the allowance or method(s) selected. A few areas where documentation is extremely important:
- Qualitative factors and forecasts should be supported by sound and reasonable documentation.
- Choice of a methodology is less important than documentation as to why the methodology chosen is appropriate.
- If using a vendor, a common pitfall is overreliance. Documentation in support of the vendor’s methodology and its appropriateness is critical. Often vendors will supply documentation in regards to their methodology; however, this should simply be a part of management’s overall policy documentation and not a substitute for it. Remember that use of a vendor does not alleviate management’s responsibility for the model.
- Use of peer data should be supported by documentation as to the appropriateness of the data and its similarity to the institution or asset segment risk profile.
With these insights in mind, let’s take a look at some helpful tips and suggestions for entities yet to adopt, with a focus on those applicable to smaller institutions given the largest institutions were among those first in line.
- Ensure the complexity of your model is in line with the complexity of your institution and the data available to support it.
- Leave appropriate time for data validation, and ensure that appropriate internal controls are in place in relation to the completeness and accuracy of the data utilized.
- Use of a vendor is not a requirement; however, vendors are often providing additional value beyond compliance with GAAP, including enhanced asset/liability management (ALM) modeling, forecasting, and other uses of data for competitive advantage.
- Anticipate that regulators will likely compare assumptions utilized in your CECL model to other areas such as your ALM modeling and stress testing. If assumptions used are dissimilar, expect to be challenged.
- Take the time now to ensure your internal controls and data governance policies are formalized and up to date, and take any actions necessary to remediate deficiencies.
- Ensure appropriate lines of communication exist between various departments involved including IT, lending, accounting, etc.
- Keep in mind that ongoing monitoring, validation, and changes in assumptions will be an important part of CECL post-adoption.
With under two years to adoption, now is the time to be planning for CECL implementation. Many useful lessons have been learned over the past year as larger institutions have adopted CECL and their methodologies have been questioned by outside stakeholders and regulators. It is important to remember that increased complexity does not always lead to the best result, especially for institutions with limited resources or reliable data. However, these limitations do not preclude an institution from reaching a reasonable, supportable, and compliant CECL methodology. Start early, document the reasoning for conclusions reached, and seek input from various stakeholders. As always, the professionals at BNN are here to help assist you as you work through the implementation of CECL at your institution.
Disclaimer of Liability: This publication is intended to provide general information to our clients and friends. It does not constitute accounting, tax, investment, or legal advice; nor is it intended to convey a thorough treatment of the subject matter.